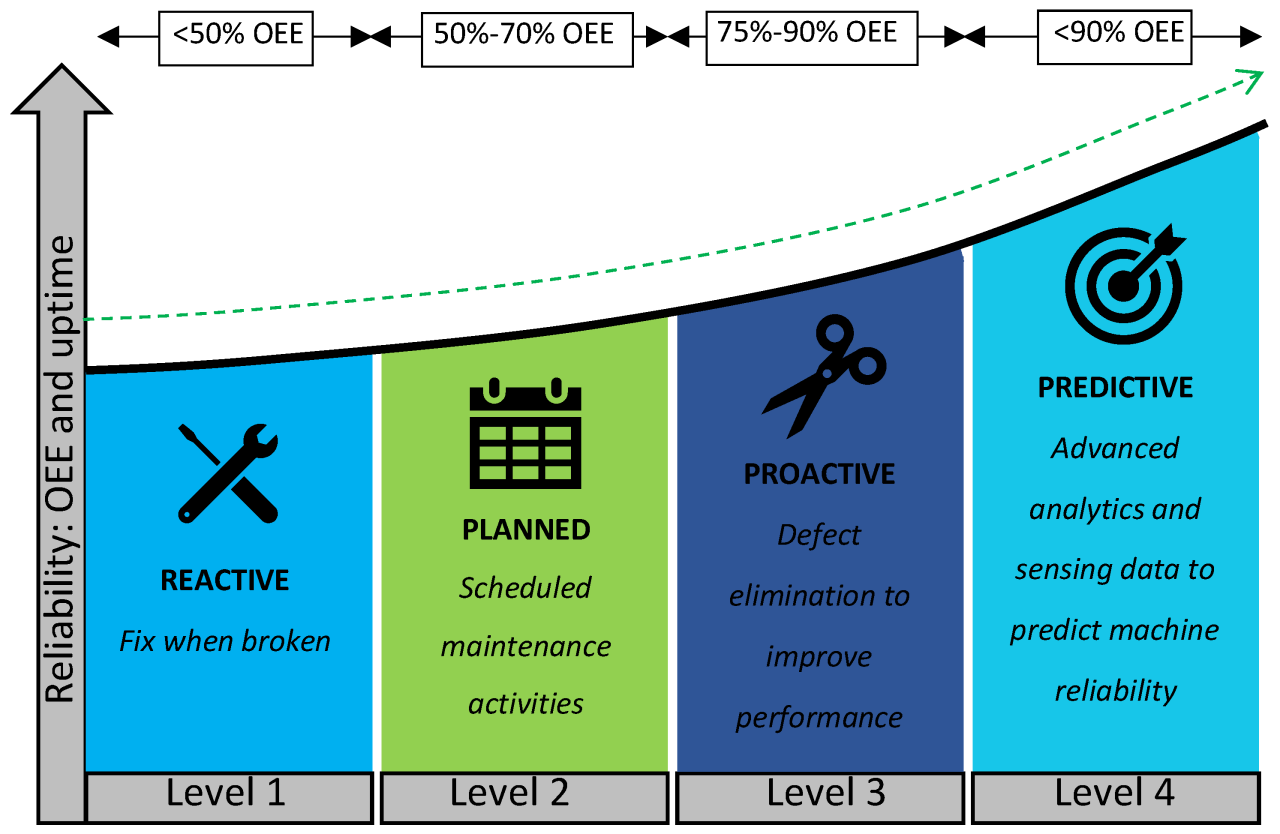
The Role of AI in Predictive Maintenance for Industrial Equipment
The Industrial Internet of Things (IIoT) has revolutionized the way industrial equipment is maintained and operated. One of the key technologies driving this transformation is Artificial Intelligence (AI), which is being increasingly used for predictive maintenance. Predictive maintenance is a proactive approach to maintenance that uses data and analytics to predict when equipment is likely to fail, allowing maintenance to be scheduled before a problem occurs. In this article, we will explore the role of AI in predictive maintenance for industrial equipment and how it is improving efficiency, reducing downtime, and increasing productivity.
The Challenges of Traditional Maintenance Approaches
Traditional maintenance approaches, such as reactive maintenance and preventive maintenance, have several limitations. Reactive maintenance, which involves fixing equipment only after it has broken down, results in costly downtime, lost productivity, and potential safety risks. Preventive maintenance, which involves regular scheduled maintenance, can be time-consuming and may not always be effective in preventing failures.
The Emergence of Predictive Maintenance
Predictive maintenance, on the other hand, uses advanced analytics and machine learning algorithms to analyze data from sensors, machines, and other sources to predict when equipment is likely to fail. This approach enables maintenance teams to schedule maintenance at a convenient time, reducing downtime and increasing productivity.
The Role of AI in Predictive Maintenance
AI plays a crucial role in predictive maintenance by analyzing large amounts of data from various sources, including:
- Sensor Data: AI can analyze data from sensors installed on equipment, such as temperature, vibration, and pressure sensors, to identify patterns and anomalies that may indicate potential failures.
- Machine Data: AI can analyze data from machines, such as production schedules, quality control data, and maintenance records, to identify trends and patterns that may indicate potential failures.
- Historical Data: AI can analyze historical data from similar equipment or machines to identify common failure patterns and trends.
AI algorithms can then use this data to:
- Identify Anomalies: AI can identify unusual patterns or anomalies in the data that may indicate potential failures.
- Predict Failures: AI can predict when equipment is likely to fail, allowing maintenance teams to schedule maintenance at a convenient time.
- Optimize Maintenance: AI can optimize maintenance schedules based on the predicted failure times, reducing downtime and increasing productivity.
Benefits of AI-Powered Predictive Maintenance
The benefits of AI-powered predictive maintenance are numerous, including:
- Reduced Downtime: Predictive maintenance reduces downtime by allowing maintenance teams to schedule maintenance at a convenient time, reducing the likelihood of unexpected failures.
- Increased Productivity: Predictive maintenance increases productivity by reducing downtime and allowing production to continue uninterrupted.
- Cost Savings: Predictive maintenance reduces costs by reducing the need for reactive maintenance, which can be expensive and time-consuming.
- Improved Safety: Predictive maintenance improves safety by reducing the risk of unexpected failures, which can pose a risk to personnel and equipment.
- Extended Equipment Life: Predictive maintenance can extend the life of equipment by identifying potential problems early, allowing maintenance teams to take corrective action.
Case Studies
Several companies have already seen significant benefits from implementing AI-powered predictive maintenance, including:
- General Electric: GE has implemented a predictive maintenance system that uses AI to analyze data from its jet engines, reducing downtime and increasing productivity.
- Siemens: Siemens has developed a predictive maintenance system that uses AI to analyze data from its wind turbines, reducing downtime and increasing energy production.
- Schneider Electric: Schneider Electric has implemented a predictive maintenance system that uses AI to analyze data from its industrial equipment, reducing downtime and increasing productivity.
Challenges and Limitations
While AI-powered predictive maintenance has many benefits, there are also several challenges and limitations, including:
- Data Quality: The quality of the data used to train AI algorithms is critical. Poor-quality data can lead to inaccurate predictions and reduced effectiveness.
- Data Integration: Integrating data from various sources, including sensors, machines, and historical data, can be challenging.
- Algorithm Complexity: AI algorithms can be complex and require significant expertise to develop and implement.
- Cost: Implementing AI-powered predictive maintenance can require significant investment in hardware, software, and personnel.
Conclusion
AI-powered predictive maintenance is revolutionizing the way industrial equipment is maintained and operated. By analyzing large amounts of data from various sources, AI algorithms can predict when equipment is likely to fail, allowing maintenance teams to schedule maintenance at a convenient time. This approach has numerous benefits, including reduced downtime, increased productivity, cost savings, improved safety, and extended equipment life. While there are challenges and limitations to implementing AI-powered predictive maintenance, the benefits far outweigh the costs. As the IIoT continues to evolve, we can expect to see even more innovative applications of AI in predictive maintenance.