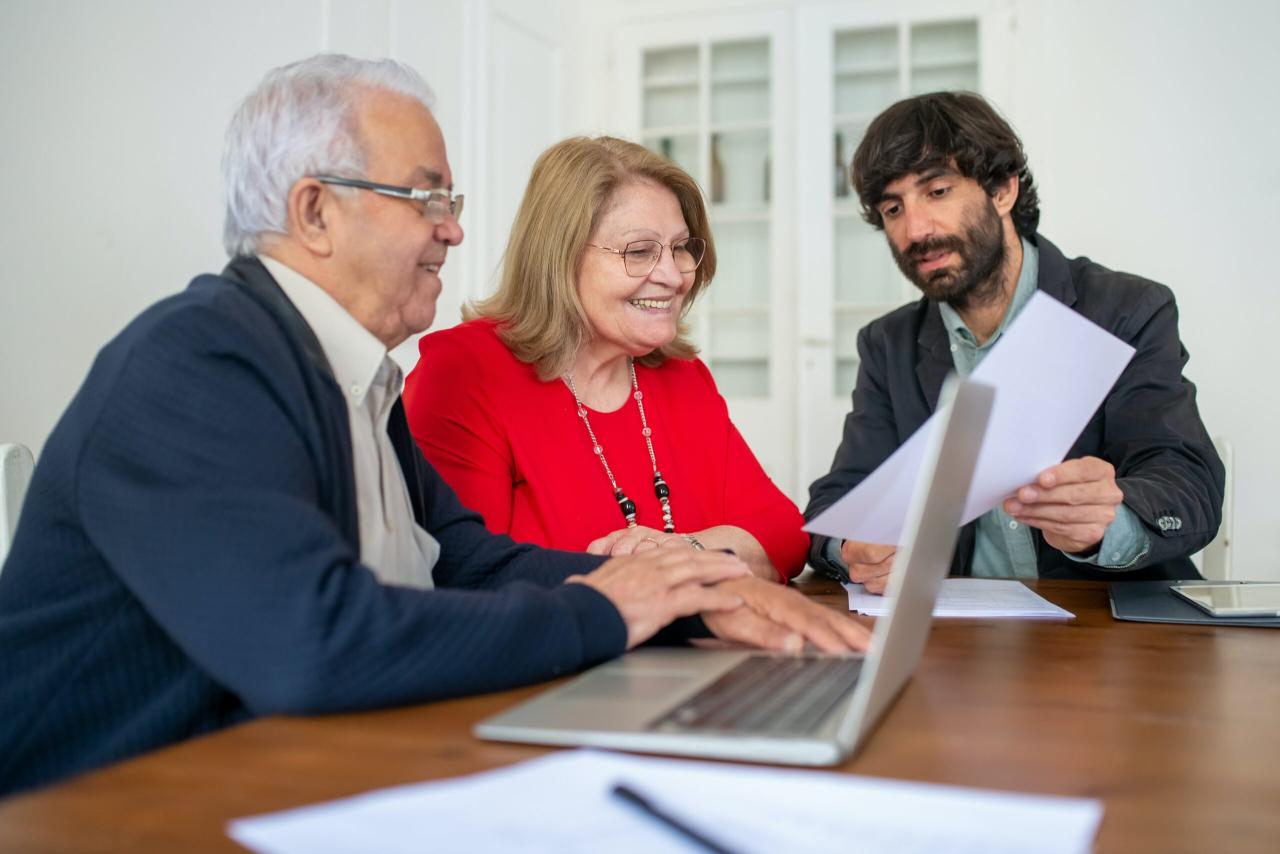
The Future of AI in Retail Analytics: Revolutionizing the Industry
The retail industry has undergone significant transformations in recent years, driven by advancements in technology and changing consumer behaviors. One of the most promising developments in this space is the integration of Artificial Intelligence (AI) in retail analytics. AI has the potential to revolutionize the way retailers operate, making them more efficient, effective, and customer-centric. In this article, we will explore the future of AI in retail analytics, its applications, benefits, and challenges.
The Current State of Retail Analytics
Retail analytics involves the use of data and analytics tools to gain insights into customer behavior, preferences, and shopping patterns. The primary goal of retail analytics is to improve business outcomes, such as increasing sales, reducing costs, and enhancing customer experience. Traditional retail analytics relies on manual data collection, analysis, and interpretation, which can be time-consuming, labor-intensive, and prone to errors.
The advent of big data, IoT sensors, and social media has led to an explosion of data, making it challenging for retailers to gather, analyze, and act upon insights in a timely manner. This is where AI comes into play, offering a solution to the complexities of retail analytics.
Applications of AI in Retail Analytics
AI in retail analytics has numerous applications, including:
- Customer Segmentation and Profiling: AI algorithms can analyze customer data, such as demographics, behavior, and preferences, to create accurate and personalized profiles. This enables retailers to tailor their marketing strategies, product offerings, and customer service to specific customer segments.
- Predictive Analytics: AI-powered predictive models can forecast sales, demand, and customer behavior, enabling retailers to optimize inventory management, supply chain logistics, and pricing strategies.
- Sentiment Analysis: AI-driven sentiment analysis can analyze customer feedback, reviews, and social media posts to gauge customer sentiment, identifying areas of improvement and opportunities for growth.
- Product Recommendation: AI-powered product recommendation engines can suggest products to customers based on their browsing history, purchase behavior, and search queries, increasing the likelihood of conversion and enhancing customer experience.
- Inventory Management: AI algorithms can analyze sales data, seasonality, and other factors to optimize inventory levels, reducing stockouts and overstocking.
- Supply Chain Optimization: AI can optimize supply chain logistics, predicting demand and streamlining transportation, warehousing, and delivery operations.
- Store Operations: AI can analyze data from IoT sensors, cameras, and other sources to optimize store layouts, inventory placement, and staff allocation.
Benefits of AI in Retail Analytics
The integration of AI in retail analytics offers numerous benefits, including:
- Improved Accuracy: AI algorithms can analyze vast amounts of data quickly and accurately, reducing errors and improving decision-making.
- Increased Efficiency: AI can automate manual tasks, such as data analysis and reporting, freeing up resources for more strategic activities.
- Enhanced Customer Experience: AI-driven personalization and product recommendation can create a more engaging and relevant shopping experience for customers.
- Competitive Advantage: Retailers that adopt AI in retail analytics can gain a competitive edge over their peers, driving business growth and profitability.
- Cost Savings: AI can help retailers reduce costs by optimizing inventory management, supply chain logistics, and store operations.
Challenges and Limitations of AI in Retail Analytics
While AI in retail analytics offers numerous benefits, it also presents several challenges and limitations, including:
- Data Quality and Integrity: AI algorithms require high-quality, accurate, and unbiased data to produce reliable insights.
- Interpretability and Transparency: AI models can be complex and difficult to interpret, making it challenging for retailers to understand the basis of AI-driven decisions.
- Explainability and Trust: Retailers may struggle to explain AI-driven decisions to stakeholders, including customers, employees, and investors.
- Data Privacy and Security: AI in retail analytics raises concerns about data privacy and security, particularly in the context of customer data.
- Talent and Skills Gap: Retailers may need to invest in training and upskilling their workforce to work effectively with AI and analytics tools.
Future Outlook and Trends
The future of AI in retail analytics looks promising, with several trends and developments shaping the industry:
- Increased Adoption: More retailers will adopt AI in retail analytics, driven by the need for competitive advantage and improved efficiency.
- Cloud-Based Solutions: Cloud-based AI solutions will become more prevalent, offering scalability, flexibility, and cost savings.
- Edge Computing: Edge computing will enable real-time processing and analysis of IoT sensor data, enhancing in-store customer experience and operations.
- Explainable AI: There will be a growing demand for explainable AI, enabling retailers to interpret and trust AI-driven decisions.
- AI-Powered Chatbots: AI-powered chatbots will become more sophisticated, enabling retailers to provide personalized customer service and support.
Conclusion
The future of AI in retail analytics is bright, with the potential to transform the industry by providing insights, improving efficiency, and enhancing customer experience. While there are challenges and limitations to be addressed, the benefits of AI in retail analytics are undeniable. As the technology continues to evolve, retailers must prioritize AI adoption, invest in upskilling their workforce, and address concerns around data privacy and security. By doing so, they can unlock the full potential of AI in retail analytics and stay ahead in an increasingly competitive market.