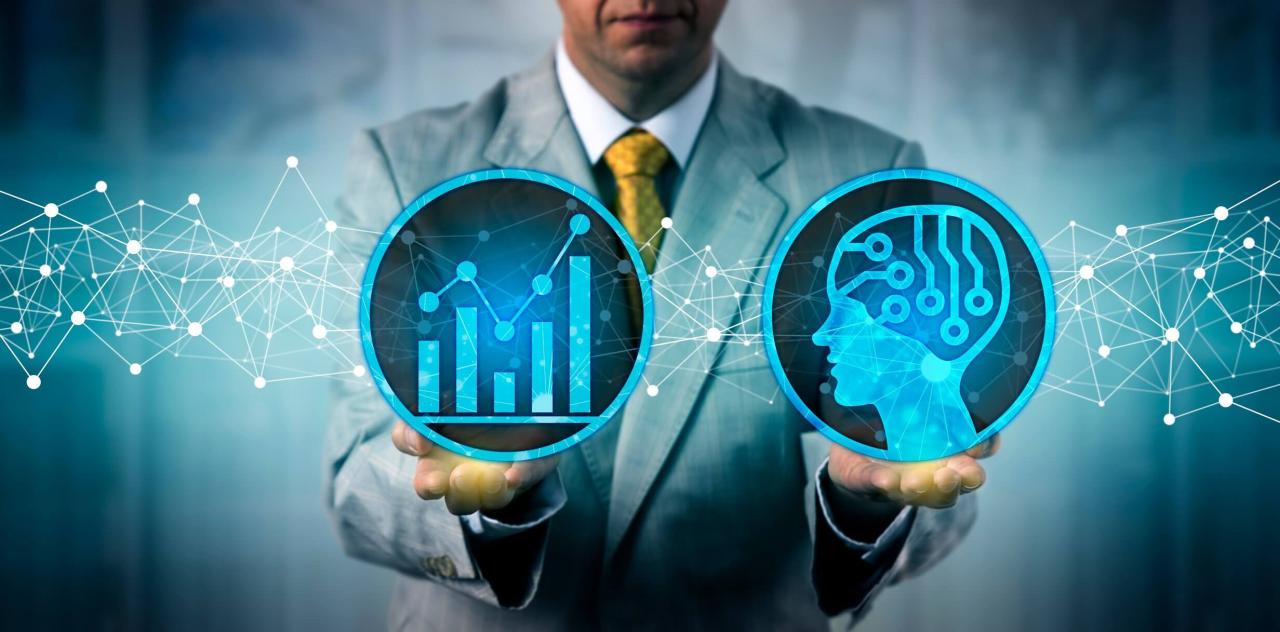
The Role of AI in Predictive Modeling: Revolutionizing Decision-Making
In today’s fast-paced and data-driven world, predictive modeling has become an essential tool for businesses, organizations, and individuals to make informed decisions. Predictive modeling involves using statistical techniques and machine learning algorithms to forecast future outcomes based on historical data. The integration of Artificial Intelligence (AI) in predictive modeling has revolutionized the field, enabling organizations to make more accurate predictions, reduce risks, and uncover new opportunities. In this article, we will explore the role of AI in predictive modeling, its benefits, and the various applications across industries.
What is Predictive Modeling?
Predictive modeling is a type of data analysis that uses statistical and mathematical techniques to predict the likelihood of an event or a behavior. It involves building a model that can identify patterns in historical data and use that information to make predictions about future events. Predictive modeling is widely used in various fields, including finance, healthcare, marketing, and sports, to name a few.
The Role of AI in Predictive Modeling
Artificial Intelligence (AI) has transformed the field of predictive modeling by introducing advanced algorithms and techniques that can analyze large amounts of data quickly and accurately. AI-based predictive models can learn from data, identify patterns, and make predictions in real-time. Some of the key ways AI is used in predictive modeling include:
- Machine Learning: Machine learning algorithms, such as neural networks and decision trees, are used to build predictive models that can learn from data and improve over time.
- Deep Learning: Deep learning algorithms, such as recurrent neural networks (RNNs) and convolutional neural networks (CNNs), are used to analyze complex data sets, such as images and videos.
- Natural Language Processing (NLP): NLP is used to analyze text data, such as customer feedback and social media posts, to gain insights and make predictions.
- Computer Vision: Computer vision is used to analyze image and video data, such as security footage and medical images, to make predictions and identify patterns.
Benefits of AI in Predictive Modeling
The integration of AI in predictive modeling has numerous benefits, including:
- Improved Accuracy: AI-based predictive models can analyze large amounts of data quickly and accurately, leading to more accurate predictions.
- Increased Efficiency: AI-based predictive models can automate the predictive modeling process, reducing the need for human intervention and increasing efficiency.
- Scalability: AI-based predictive models can handle large amounts of data and scale to meet the needs of growing organizations.
- Real-time Insights: AI-based predictive models can provide real-time insights, enabling organizations to respond quickly to changing market conditions.
Applications of AI in Predictive Modeling
The applications of AI in predictive modeling are vast and varied, including:
- Finance: AI-based predictive models are used in finance to predict stock prices, manage risk, and identify investment opportunities.
- Healthcare: AI-based predictive models are used in healthcare to predict patient outcomes, diagnose diseases, and develop personalized treatment plans.
- Marketing: AI-based predictive models are used in marketing to predict customer behavior, optimize marketing campaigns, and improve customer retention.
- Sports: AI-based predictive models are used in sports to predict game outcomes, optimize team performance, and identify new talent.
Challenges of AI in Predictive Modeling
While AI has revolutionized the field of predictive modeling, there are still some challenges that need to be addressed, including:
- Data Quality: AI-based predictive models are only as good as the data they are trained on, so data quality is critical.
- Explainability: AI-based predictive models can be complex and difficult to interpret, making it challenging to understand how they arrived at a particular prediction.
- Bias: AI-based predictive models can be biased if they are trained on biased data, leading to inaccurate predictions.
Conclusion
In conclusion, the role of AI in predictive modeling has revolutionized the field, enabling organizations to make more accurate predictions, reduce risks, and uncover new opportunities. The benefits of AI in predictive modeling, including improved accuracy, increased efficiency, scalability, and real-time insights, make it an essential tool for businesses, organizations, and individuals. While there are still challenges that need to be addressed, the potential of AI in predictive modeling is vast and varied, with applications across industries. As the field continues to evolve, we can expect to see even more innovative applications of AI in predictive modeling.